- Highline =>Spotting Opportunities in High Growth Markets.
- Posts
- #7 Deepfake Detection
#7 Deepfake Detection
The Next Cyber Secruity Battleground

Podcast Link:https://open.spotify.com/show/2gwZ88PmWkzDW9dC9bosy1
Top Ideas From The Podcast:
What are Deepfakes?
What Enabled the Rise of Deepfakes on the Internet?
How Could We Have Predicted the Problems Deepfakes Would Cause?
MiniBit (See our Framework in Action)

David Beckham Deepfake Example
Deep Fakes: The Next Cybersecurity Battleground
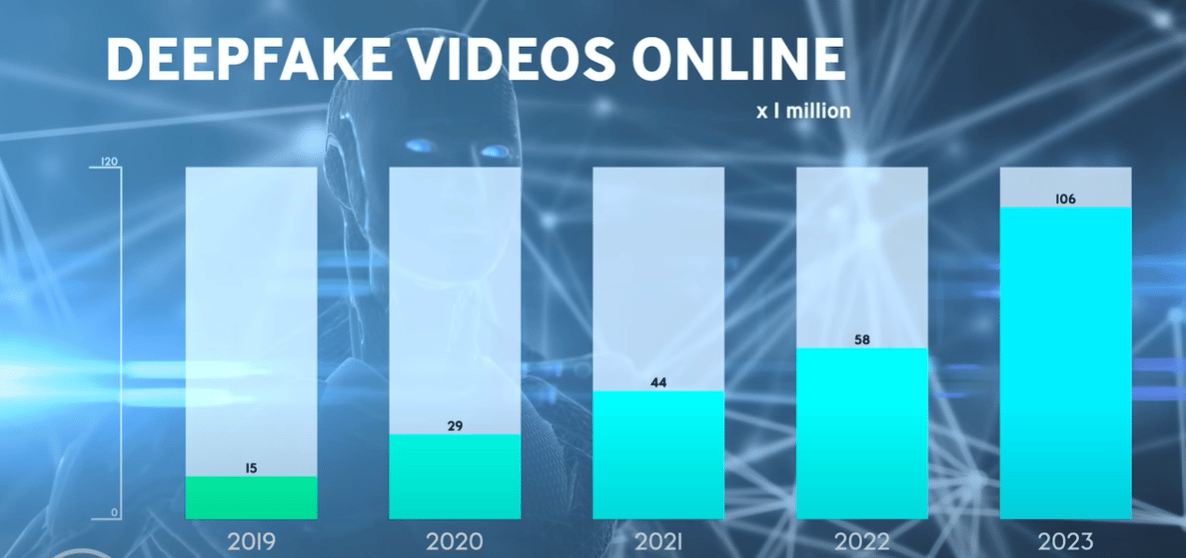
The Exponential Rise of Deepfakes on the Internet
Deepfakes are synthetic media created using machine learning. The presence of deepfakes is exponentially growing on the internet. This new form of media is being used in malicious ways by criminals and deepfake creators.
In Hong Kong, a branch manager was conned into transferring $35 million due to a deepfake impersonation of his company director's voice and corroborating fake emails. In the realm of public influence, a deepfake of David Beckham showcased him speaking nine languages fluently for a Malaria awareness campaign, demonstrating the technology's potential for positive impact when used ethically.These examples highlight deepfakes' dual potential for both sophisticated deception and innovative communication.
Watch the David Beckham DeepFake:https://www.youtube.com/watch?v=QiiSAvKJIHo
🎯How Could We Know DeepFake Detection was Going to be a Problem?
Neural networks have been around for decades, but recent access to vast online data and affordable computing power has greatly enhanced the creation of deepfakes. Cloud computing provides the necessary infrastructure and advanced chips at a lower cost, facilitating the production of highly realistic deepfakes. These can be rapidly disseminated across platforms with billions of users, raising concerns about their potential for malicious use and the significant challenges they pose to individuals, corporations, and governments due to their scalability and sophistication.
FutureSight Lens: Spotting and Assessing the Impact of Emerging Tech
The FutureSight Framework encapsulates a strategy to navigate and capitalize on emerging technologies, like deep fakes:
Spot the Game Changers: Synthetic media created using machine learning is a clear leap in quality compared to existing solutions like CGI.
Get to the Basics: Understand the fundamental shifts enabling these advancements—machine learning (ML) as the basis, bolstered by open-source data, scalable cloud computing power, and affordable advanced chips.
Think Scale: Recognise the infrastructure that facilitates the mass production of deepfakes and their proliferation on the internet, indicated by the increasing online presence of such content.
See the Big Picture: Acknowledge how deep fakes can be used for malicious intent, creating a risk for companies and governments.
Predict the Path Ahead: By applying. With this framework, we could have predicted that deep fake detection would be a thing.
Summary: Deepfakes represent a significant technical advancement rooted in core innovations that enable scalability. Understanding these technologies is crucial for early positioning in the face of their potential to become pervasive. This framework will hopefully enable us to see it early.
Podcast Link:https://open.spotify.com/show/2gwZ88PmWkzDW9dC9bosy1
MiniBit:
Here is an example of our futuresight framework in action. This is a transcript from an interview with Jensen Huang, the CEO of NVIDIA. This excerpt explains the first time Jensen saw deep learning technology (machine learning), he applied a similar framework to the futuresight framework. NVIDIA created the graphic cards (GPUs) used to train machine learning models.
“When we saw deep learning, when we saw AlexNet and realized its incredible effectiveness and computer vision, we had the good sense, if you will, to go back to first principles and ask, what is it about this thing that made it so successful?
When a new software technology or a new algorithm comes along and somehow leapfrogs 30 years of computer vision work, you have to take a step back and ask yourself, but why? Fundamentally, is it scalable? And if it’s scalable, what other problems can it solve?
There were several observations that we made. The first observation is that if you have a whole lot of example data, you could teach this function to make predictions.
What we’ve basically done is discovered a universal function approximator, because the dimensionality could be as high as you wanted to be. Because each layer is trained one layer at a time, there’s no reason why you can’t make very, very deep neural networks.”